After the previous chapters of a coffee with IoT(Chapter 0: A recipe for internal hacktivism, Chapter 1: NiFi's streams, Chapter 2: The raw data and Chapter 3: Seeing all the data in a graph) today we will talk about centralization measures in descriptive statistics.
The negotiator
Despite the non-negotiation policy, it has taken the mediation of Danny Roman and Chris Sabian to establish proper communication with the group of hacktivists addicted to condensed milk. The only statement we have been able to get has been:
"Never say no to someone who's holding hostages! It's in the manual!"
Until now, the presence of hostages was totally unknown, and this statement has caused a scandal among the journalists present.
The negotiator has arrived on the scene, madam! We'll negotiate your mortgage, we'll negotiate your divorce, we'll negotiate whatever it takes. The negotiator......! The negotiator has entered the scene!
A not-so-pretty graphic
As we saw in the previous article, it is clear that assimilating more than 5 million pieces of data at a single glance is complicated. So, it is time to resort to a tool that allows us to understand the data we have in our hands: statistics.
Moreover, there is a specific part of statistics that specializes in helping to understand a set of data: descriptive statistics. A branch of statistics that is responsible for providing summaries of data sets, both graphical and numerical, with which to address the general understanding of them.
So, today we will focus on the numerical part of descriptive statistics, i.e. the descriptive statistical parameters.
Descriptive statistical parameters
Descriptive statistical parameters are usually classified according to the type of information they provide on the set of data studied. The most frequent aspects of interest are the following:
- Centrality: Representative values of the set around which the data are grouped.
- Dispersion: A measure of how varied the data is, indicating how much the data is concentrated around the center.
- Position: Values that divide the sorted data into groups with the same number of observations.
In addition, there are other secondary categories, such as shape statistics or proportion statistics. But today we will focus on the first type of them, the centrality parameters.
*STA- and *GERBH-
The Indo-European root *sta- means 'to stand', and we can find it in words like system, institute, epistemology, destiny, state, substance, statue, fabric, static, solstice, witness, restore, metastasis, resistance, establish, station, noun, stable, insist, stature, institution, stamen, restaurant, obstinate, exist, armistice, post or banner. Of course, it is also found in statistics, since this branch of mathematics was born as the science of the state, which was also called political arithmetic.
For its part, the root *gerbh-, which means 'scratch' or 'scratch', takes us to the origins of writing, to a time when drawings were becoming letters, as it refers to the way of printing marks on clay, to write or draw symbols. Thus, we can find this root, transformed into the Greek root *gra- (γρά), in the words grammar and graph, as well as in demography, graph, autograph, crossword, pentagram, pen...
By the way, there are some discrepancies about the origin of writing(*gerbh-), but it is almost certain that if you read up on the subject you will find a story that it is related to tax collection or trade between states(*sta-). One of the most common ones refers to the fact that writing replaced an earlier system used for counting cattle and based on clay spheres called, coincidentally, calculi (that's why counting and kidney stones are so similar).
Centralization measures
The descriptive statistical parameters of centralization allow to obtain a value that represents all the data, calculating, by different methods, the center of the studied values:
- Average: 70.96772 watts
We normally identify it with the arithmetic mean, although there are other possible means (harmonic, quadratic, geometric...). In this way, it is calculated as the quotient between the sum of all the data and the amount of observed data.
It is related to the mathematical hope, which is the tendency of the results of an experiment when it is repeated a large number of times. For example, the hope when rolling a 6-sided die is 3.5 (the sum of the values of all its faces divided by the number of faces of the die):
1 + 2 + 3 + 4 + 5 + 66= 3.5
An interesting case, in which the value used to summarize the data turns out to be a value impossible to find in the data itself (we cannot obtain a 3.5 in the roll of a die).
It is also related to the center of mass of an object, which is relevant, for example, in the calculation of architectural structures. But I'll leave that to my past self.
- Median: 32.34943 watts
The median literally refers to what is in the middle. It is calculated by ordering the values from smallest to largest, and taking the one that is in the central position. It is, therefore, the value at which half of the observations are above the median . Or in other words, that value that separates half of the lowest data from half of the highest data.
If the mean is the most famous and used statistical value (although less well understood), the median is the most forgotten. However, it has a very interesting property that makes it very attractive for statisticians: robustness. A property thanks to which it is often preferable to use the median instead of the mean. Let's see this concept with an example.
Let's imagine an ordered list with numbers from 0 to 100:
0,1,2,3,4,5,…,96,97,98,99,100
Both its mean and median are worth 50. Now, let's change the value of one of the numbers. For example, the number 25 becomes 26, so we have two 26s and no 25s:
0,1,2,3,4,5,…,23,24,26,26,27,28,…,96,97,98,99,100
Now our median is still 50, since it's still the number in the middle of the list, but the mean has changed a little bit, and it's now 50.01. Let's go further. Let's say we change the 100 to the number 1,000,000:
0,1,2,3,4,5,…,96,97,98,99,1.000.000
In this case, we would still have the number 50 in the central position (median), but our mean would be 10,049. We can see that the value of the mean changes with the change of only one data. On the other hand, the median can resist the change of up to half of the data. This ability is called robustness, and it has an enormous relevance, especially in the construction of predictive models.
- Fashion: 0 watts
The mode is the third statistic of central tendency that is generally known (it is part of the compulsory education syllabus). It is obtained simply by determining the most frequent value of a variable and is possibly the most peculiar of the three.
In order to calculate it, it is necessary that the variable under study has defined values that can be repeated a certain number of times. Therefore, it cannot always be calculated for numerical variables. Although, at the same time, this allows it to be calculated for categorical variables (colour of cars, clothing sizes...). It is the only centrality statistic that, when calculated, can have no value, only one or several. Thus, there are cases in which there are several fashions when different values are repeated the same number of times. While we have no mode if all values are repeated the same number of times. Finally, it should be noted that the mode can be very far from the center of a distribution (as in our data, whose mode is 0).
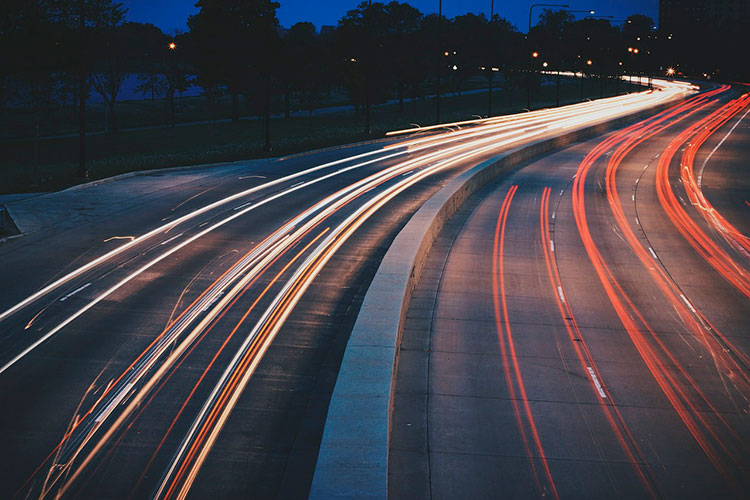
On Man and the Development of the Human Faculties: An Essay on Social Physics
1835, Adolphe Quetelet, one of the fathers of statistics.
So, with the data of centrality of the electrical consumption of the coffee machine, we can say that the amount of watts (measured with a precision of 5 decimals) that the machine most usually consumes is 0 watts, although we can expect that its general consumption is 70.96772 watts, although its typical consumption is around 32.34943 watts.
Or to put it another way.
If the consumption of the machine were lottery numbers, the best option is to bet on 0, since there are more balls with this number in the draw drum than with any other.
If, on the other hand, we want to forecast the cost of the machine's electricity consumption bill, we must assume that it continuously consumes 70.96772 watts.
Finally, if we were in a contest in which we had to predict the value of electricity consumption at a given time, and they were taking money away from the prize in relation to the error made in the prediction, we would arrive with more money at the end of the contest if we always say 32.34943 watts, that is, if we give as an answer in all rounds the median, instead of giving the mean or mode.
What other descriptive statistical parameters can we use to know the electrical consumption of the coffee machine?
We will see this in the following deliveries of IOT coffee